Why Data Scientists Are Eyeing the Exit
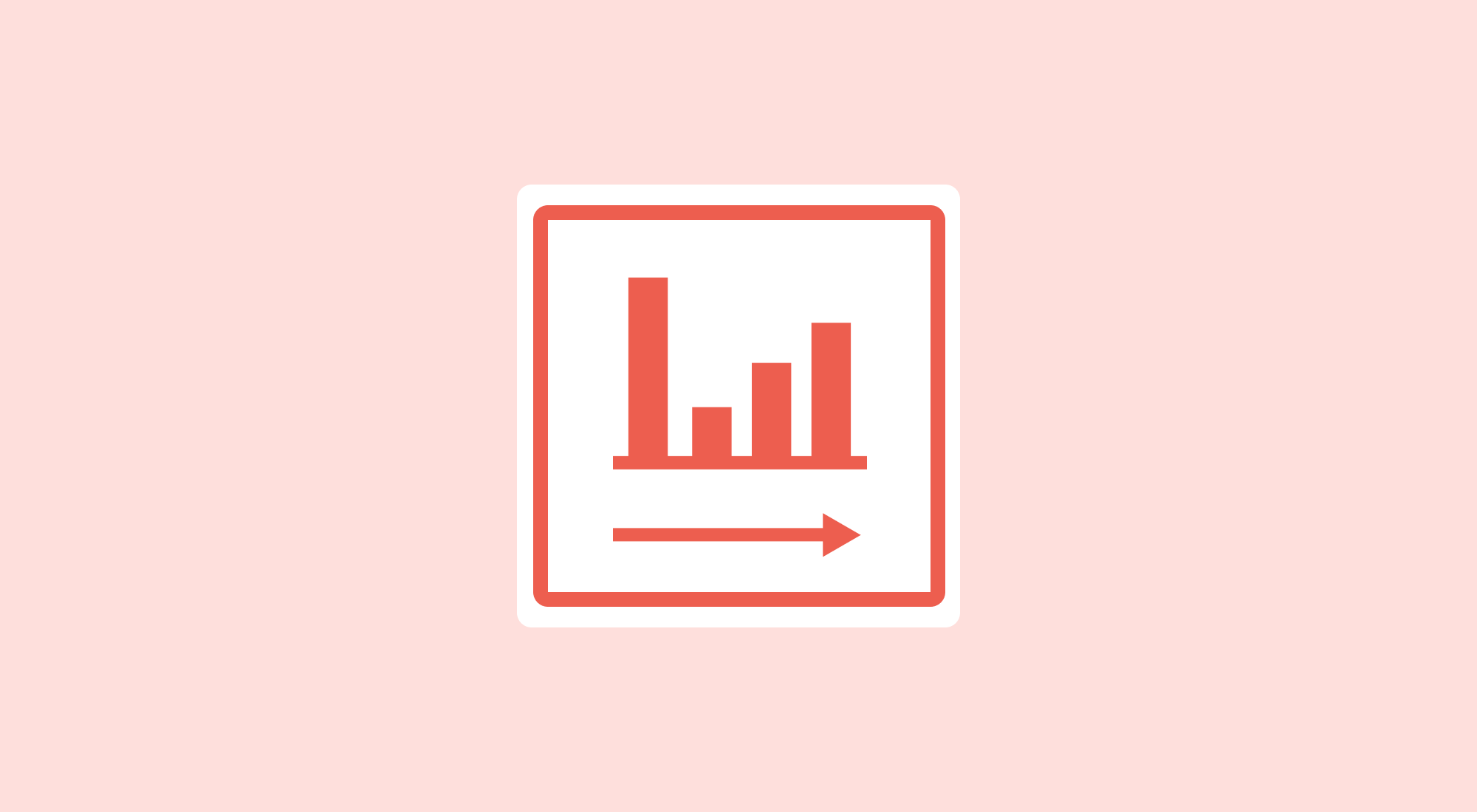
‘Data scientist’ was named the sexiest job of the 21 century. The best job in America boasts some of the highest starting salaries of any entry-level role and are in extremely high demand (There’s a shortage of 151,717 data scientists in the U.S. alone).
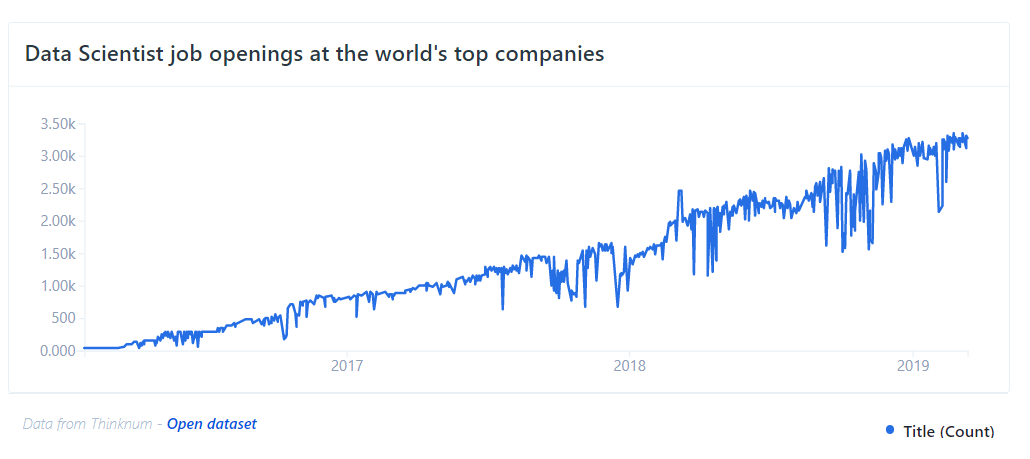
In addition to the sky-high salaries, top tier companies like Microsoft, Apple, Google, Amazon, IBM, and Facebook that are eager to shower them with incredible perks like free gourmet meals, nap pods, spa treatments, laundry service, and even complimentary access to the company yacht — in case they ever want to take a break from crunching numbers and go out for a pleasure cruise. On top of all that, they get to work on work on challenging problems and use interesting technologies.
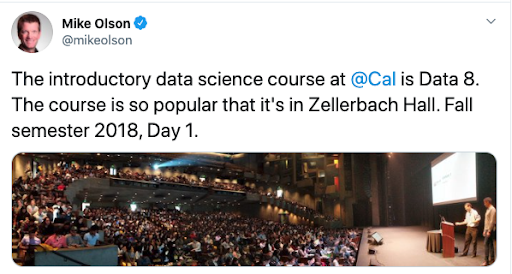
But despite all of this upside, 65% of data scientists are unhappy in their current roles and looking for a way out. Half plan to leave their companies this year. Additionally, Google Trends data shows the phrase “I hate data science” has been gradually rising since 2019 – further indication of the growing discontent amongst data scientists.

So what’s going on? Why do so many data scientists want out of their roles?
3 reasons data scientists are frustrated
They hate what they are asked to do most
In school, budding data scientists envision their future roles doing exciting and impactful work like building sophisticated machine learning algorithms that surface insights to top executives.
However, once in the role, data scientists face a harsh reality. 80% of their time is spent cleaning and organizing data – manual, tedious work that’s far from glamorous. 76% of data scientists say this is the least enjoyable part of the job.
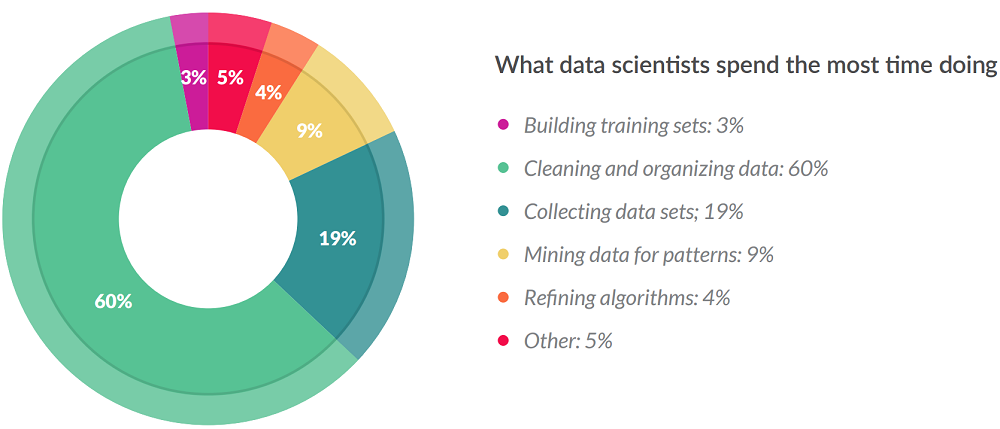
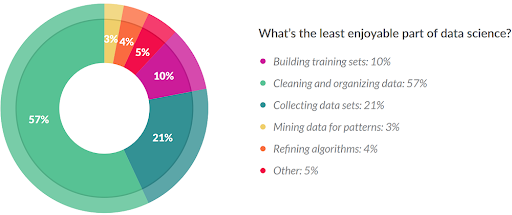
The tedium of cleaning and organizing data is exacerbated by sheer volume of data in use. According to our survey Bridging the Gap Between Data and Business Teams, 25% of data experts cite slow SQL as the main bottleneck to reporting while 27% blame large and unwieldy data sets.
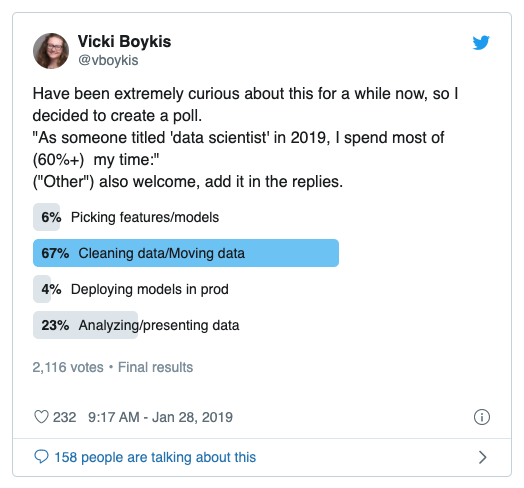
But “munging” dirty and disorganized data isn’t the only thing sucking up their time and preventing them from doing the work they’re best at.
They’re stuck in report factory hell
The precious little time that’s left over after getting data is cleaned and organized is often quickly sucked by low-level data requests. More than three quarters of data experts report that up to 49% of their or their team’s time is spent preparing ad-hoc reports for line of business needs.
Ad-hoc is Latin for “as the occasion requires,” but dynamic businesses are ad hoc by nature. When competing requests pour in, data teams are flooded with tedious, time consuming, and often duplicate work. Once the reports are submitted, the work is often far from over. Data teams receive and average of 3 to 4 follow up questions.
It’s called report factory hell – an endless loop of creation, presentation, and iteration.
Even worse, these low level reports add little value to the org as a whole, leaving data teams with minimal time and resources to do broader, more strategic work with farther reaching impact.
They’re misunderstood
Much of that misalignment between the work they do and the organization’s goals is due to the “data language barrier.” Business leaders have lofty expectations for data science. They envision receiving clearcut insights that revolutionize the business by shaping goals and revealing insights into customer behavior and needs. But they don’t realize it’s a collaborative process that requires their domain expertise. Business leaders can have an incomplete understanding of the way data is collected, organized, and analyzed. They make assumptions about how much the data team knows and what data they have access to.
Instead, they take a waterfall approach — chucking data requests over the wall and leaving data teams to do the heavy lifting. Data experts are left having to define the problem as well as work out the solution with limited insights into the nuances of the issue, undefined guidelines, and vague goals. The assumption is that they don’t need support or collaboration from other teams to do their work.
Half of data experts admitted they struggle to interpret domain experts’ data questions or needs. Without that domain expertise and with the parameters of problems hazy in their minds, the solutions data scientists come up with are educated guesses at best. This process of trial and error takes several months to arrive at the desired outcome, which delays the business requirements in due process. After endless back and forth trying to meet the needs of their business colleagues, the result is often disappointment. Business users struggle to see any tangible benefits or return on the organization’s investment in data science. It’s estimated that 85% of big data projects end in failure — misalignment with business goals and objectives being a key factor.
These 3 factors aren’t anticipated by many who enter the field with big dreams. All too often, the sobering reality of the job extinguishes their passion for data.
Put the Spark back into Data Science
It doesn’t have to be this way. In uncertain times like ours, data is more crucial than ever. Company leaders need to make sound decisions and data has the power to help light the path forward.
Organizations looking to retain their top data talent and get more value out of their data need to quickly take action in 3 area
Improve data literacy to get everyone speaking the same language
Build confidence and improve data literacy by holding training sessions and workshops. Instead of making assumptions, conduct an internal poll to accurately determine where knowledge gaps are so you can tailor training programs to address the needs of your team
Business users in particular need to be educated on what data is available, what is possible and what isn’t. Host workshops, lunch and learns, and mixed team exercises to break down communication silos and get people working together. When both groups of people can share their expertise on an equal footing, respect and empathy grow.
Free data scientists to put their skills to best use
Empowering business users helps unburden data experts to escape report factory hell. Modern data analytics tools like Sigma have helped data experts free up to 50% of their time by minimizing ad-hoc requests and endless back and forth. This enables data scientists to do the work they’re best at and find most rewarding.
Modern analytics tools like Sigma sit on top of your cloud data warehouse so the data is always fresh and can be customized to fit any team’s needs. This enables business users to freely go beyond the dashboard and explore the underlying data at will without compromising security.
Take a community-driven approach to analytics
Data is a team sport and should be community-driven for the best results. The good news is that a whopping 79% of data experts and 64% of business experts desire to work together more closely.
When everyone can share and build on each other’s work, innovation prevails and people are able to use their expertise most effectively. The humble spreadsheet has proven itself to be the preferred data insights conduit for both business and data experts, with 85% of people using spreadsheets in their work. And even amongst people that understand and use SQL, 88% still turn to a spreadsheet when exploring data. Modern BI tools like Sigma enable true collaboration between data and domain experts through features like collaborative data modeling, a common spreadsheet interface, the ability to write views back to the data warehouse for use across the full data ecosystem, and more.
Data Shows the Future is Bright for Data Scientists
Despite the challenges, the future looks bright for data scientists. The role is projected to boom in the next decade. Organizations are collecting more data than ever before, and the need for talented and creative problem solvers to help them surface actionable insights has never been greater.
Putting those creative problem solving skills to use improving data literacy, adopting tools that enable collaboration, and taking a more community-driven approach to analytics will be key to ensuring data science continues to be a lucrative and rewarding career for decades to come.
Let’s Sigma together! Schedule a demo today.