Identify Your Highest Value Shoppers for Maximum Profitability
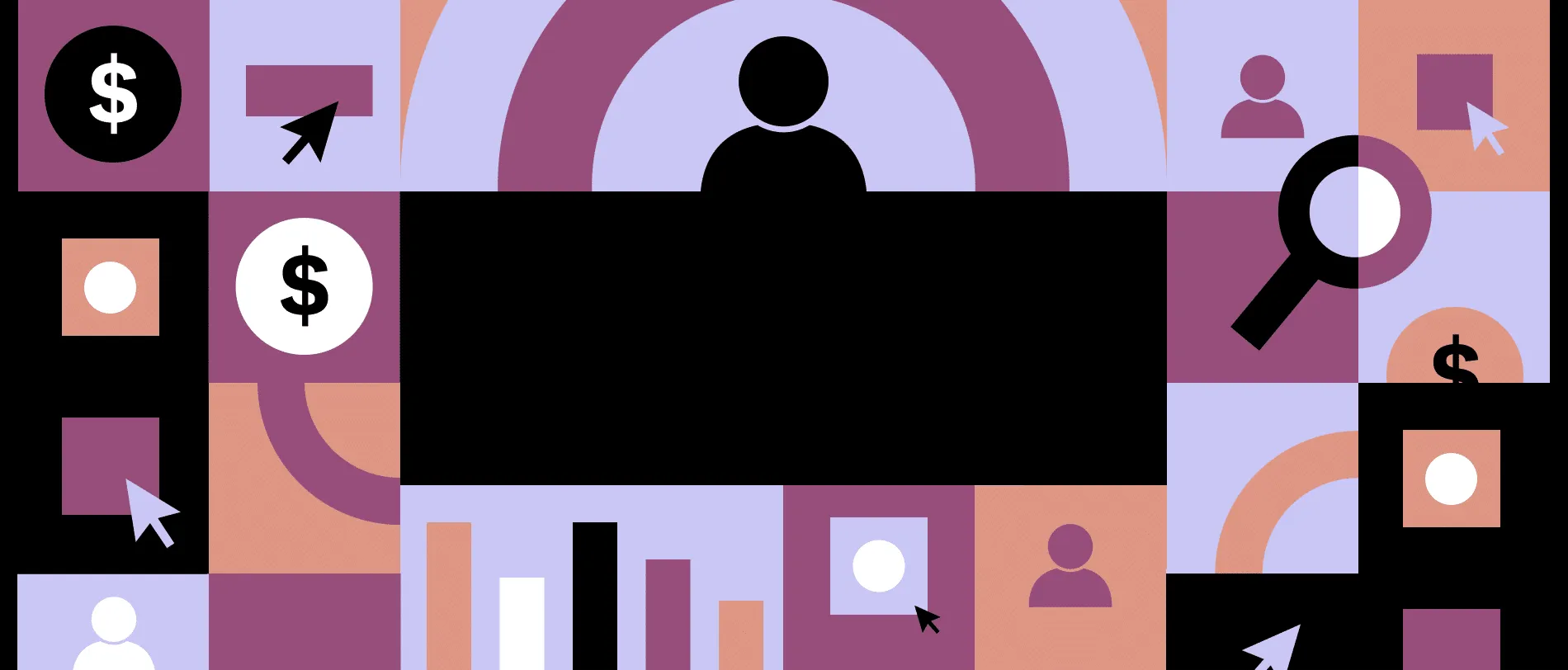
Target segment analysis is the process of dividing customers into groups, or cohorts, with similar attributes and purchase behaviors to determine which are the most profitable. Marketing campaigns, product mixes, and recommendations can then be tailored to these specific segments, improving basket sizes, acquisition costs, and lifetime value.
When to consider this play
- You need to identify, refine, or expand your target market.
- You’re trying to determine or update your ideal product mix or assortment.
- You want to make more relevant product recommendations.
- Your company runs dozens, hundreds, or even thousands of campaigns across multiple channels and needs to optimize targeting, budget, and messaging.
- You need to more accurately forecast profit and predict outcomes.
- You’re looking to help maximize shopper retention and lifetime value.
Goals
- Determine who your top customers are
- Identify common traits and behaviors for your most valuable shoppers
- Maximize campaign conversion rates while minimizing acquisition costs
- Accurately anticipate and deliver on forecasts
- Identify niche or untapped markets
- Optimize product mix to attract and convert high-value customers
- Increase cross-sells and upsells through better recommendations
- Improve customer experiences to nurture loyalty and lifetime value
Play Prep
DATA NEEDED
Point-of-Sale (POS) data – Clover, Square, etc.
Ecommerce app data – Shopify, Lightspeed, etc.
Inventory information – QuickBooks, NetSuite, etc.
Website analytics – Google Analytics, Kissmetrics, etc.
CRM data – Salesforce, Pipedrive, etc.
Social media data – Twitter, LinkedIn, etc.
Email analytics – HubSpot, Campaign Monitor, etc.
Ad data – Facebook Ads, Google Ads, etc.
KEY PLAYERS
Merchandising
Inventory management
Data analysis for product/merchandising
Digital marketing
Retail sales
Product manager of growth
Executing the Play
Step 1: Establish a single source of truth
Step 2: Collect the right shopper data
Step 3: Integrate your data for a complete 360 degree view
Step 4: Identify core metrics with dollar-based outcomes
Step 5: Get comfortable with cohort analysis
Step 6: Optimize your marketing and merchandising efforts
1. Establish a single source of truth
Individual retail tools like eCommerce applications, inventory management platforms, website analytics solutions, and POS systems represent narrow, siloed slices of the customer journey. To get a full picture of customers across each of these touch points and measure impact all the way from first touch through retention, you must have a single, centralized repository where all of your data exists.
Start by asking your data or BI team if you have a cloud data platform (CDP) — also known as a cloud data warehouse — and whether the data sources listed in the Play Prep section of this playbook are being pulled in.
WE DO NOT HAVE A CLOUD DATA PLATFORM
Talk to your BI team about implementing one. Solutions like the Snowflake Data Cloud, Amazon Redshift, and Google Big Query leverage the unlimited scale and speed of the cloud, making it easy to store, manage, and analyze massive volumes of live data from across sources.
WE HAVE CLOUD DATA PLATFORM BUT SOME OF MY DATA SOURCES ARE NOT IN IT
Simply ask your BI team to start integrating your key desired data sources into your cloud data platform so they can more easily be analyzed together to generate insights. Since cloud data platforms are elastic, can handle all types of data, and separate data storage and compute costs, this shouldn’t be an issue. Pre-built data connectors from companies like Fivetran can also help.
WE HAVE A CLOUD DATA PLATFORM AND ALL OF MY DATA SOURCES ARE IN IT
Wonderful — proceed to Step 2.
2. Collect the right shopper data
To identify and understand your top targets, you need to collect as much information about them as possible as they move through the purchase funnel. Once you have a single source of truth, you can focus on capturing the shopper traits and behaviors your business needs to effectively reach and convert different audience segments.
It’s likely you won’t have all of the data you’d like, but companies like GravyAnalytics and Zoominfo can help enrich datasets to better understand your customers and the shoppers engaging with your marketing initiatives.
Depending on your business model, customers, and campaigns, some types of information are more helpful for segmentation than others:
TYPES OF DATA FOR SEGMENTATION
Demographic – Demographic segmentation is one of the most basic and common types of market segmentation and includes grouping audiences by things like age, sex, marital status, family size, occupation, education level, income, race, nationality, and religion.
Psychographic – Psychographic segmentation groups audiences by personal motivations, beliefs, lifestyles, personality traits, values, opinions, and interests. This can be helpful if your target market is very large and broad.
Geographic – Geographic segmentation is a subdivision of demographic segmentation and groups audiences by city, state, country, zip code, or other regional classification.
Behavior – Behavioral segmentation groups audiences by common behaviors including purchase behaviors, content consumption, engagement, and more.
3. Integrate your data for a complete
360 degree view
While all of your data may reside in your cloud data platform, it still has to be effectively normalized and joined together for analysis. And analyzing multiple data types formatted differently across dozens of sources can be a challenge — especially when it comes to managing the types of semi-structured data created by most web applications.
Merchandisers and marketers usually take one of the following 3 approaches, each with its own considerations:
EXTRACTING DATA TO SPREADSHEETS FOR MERGING AND MANIPULATION
Most retail solutions allow the user to export data in spreadsheet formats that can be merged together for analysis. In addition, marketers and merchandisers often ask their data and BI team to extract data from the cloud data platform so they can manipulate it.
Key considerations
- Large datasets with a million+ rows created by shopper interactions across product lines and marketing campaigns cause spreadsheets to become extremely slow or crash. Many times the data is reduced to a subset to fit.
- Granular data like individual SKUs or transactions will not be accessible if the data has been reduced to a subset.
- Each retail platform formats data differently, so significant normalization must take place before it can be effectively merged together.
- Downloaded data is not real-time, so insights quickly go stale and must be updated on a regular basis.
- Manual merging and frequent updates increase the likelihood of errors and inconsistencies.
- Extracts cause data silos and varying versions of the “truth,” while sharing these reports creates version control issues, as well as security and governance risks.
DATA INTEGRATIONS AND APIS
Most marketing and merchandising solutions have integrations or APIs that hook directly into other popular tools like Salesforce or Google Analytics. Data is automatically passed between these systems and integrated together for easier analysis. Many enterprises also invest in an MDM (master data management) system or dedicated database to house and connect customer data from across sources.
Key considerations
- Eliminates some of the manual work associated with joining data, saving time and reducing errors along the way. However, data and BI teams still need to do some level of transformation to bring all this data together for analysis.
- MDM systems often take years to build out correctly, are difficult to maintain, and are prone to inaccurate insights. Adding new data sources is also a slow process that inhibits business agility.
- Many applications are not supported via integrations, so data must still be manually joined via spreadsheet or integrated and extracted from the cloud data platform by the BI team — both of which are time-consuming processes that lead to governance, scalability, and data quality issues.
- There are usually limitations around what data can be pulled in via API and how it can be manipulated.
- Integrated data is often available in aggregate only and cannot be drilled into at a granular level.
CLOUD DATA EXPLORATION AND BUSINESS INTELLIGENCE (BI) SOLUTIONS
Unlike traditional analytics tools, cloud-native analytics and BI solutions empower line of business teams like marketing and merchandising to easily access, integrate, and analyze data at the same level as data and BI experts — no coding or technical expertise required.
Key considerations
- Data is accessed directly from the cloud data platform — your company’s single source of truth for data — and no data extracts means data is always complete, fresh, and accurate.
- Eliminate the need for data or coding expertise by adopting the form and function of tools business teams are already familiar with, like spreadsheets.
- Combining data from dozens of sources into one holistic view can be done in hours instead of weeks.
- It’s possible to analyze billions of rows of data at once without hitting scale limitations.
- Data is available and accessible at the lowest level of detail for granular insights — think individual SKU and customer ID level.
- Analyses are done once, saved, and automatically updated. This makes it safe to share or reuse them and eliminates version control issues and data silos.
- It’s fast and easy to add new data sources and adapt analyses as consumer preferences, products, and marketing channels evolve over time.
- Give marketers and merchandisers the power to create rich, interactive dashboards themselves and/or drill down into the underlying data to ask follow up questions.
4. Identify core metrics with dollar-based outcomes
Retail marketers and merchandisers have many metrics they use to indicate the success of particular approaches or programs: page views, ad clicks, social media likes, email open rates, etc. However, target segment analysis must be grounded in metrics that go beyond shopper engagement and tie performance directly to dollar-based outcomes.
It’s critical for the entire business to align on these metrics and what constitutes a high-value cohort. These metrics will vary depending on your business type and goals (i.e. attracting new shoppers versus driving upsells), but some common examples include:
- Customer acquisition cost (CAC)
- Customer lifetime value (LTV)
- Basket size
- Average order value (AOV)
- Net profit margin
- % of product returned
5. Get comfortable with cohort analysis
Cohort: A group of people that share common characteristics, behaviors, and/or experiences.
Cohort Analysis: An analysis technique that divides data sets with information about shoppers or customers into smaller subsets based on shared characteristics, behaviors, and/or experiences within a given timeframe or funnel.
We recommend teams looking to identify their highest value targets start their cohort analysis by grouping users according to the metrics they identified in the previous step — e.g. by acquisition cost, lifetime value, etc.
Cohort analysis is extremely powerful, but the accuracy and insightfulness of the results depend largely on the tool teams use to conduct the analysis. For maximum efficiencies and best results, use a modern, cloud-native analytics and business intelligence solution that approaches cohort analysis with the following 3 capabilities in mind:
- SPEED. Insights are only as good as they are recent. Cohort analyses should be done querying live data, not data extracts, to provide the most recent and valuable updates. This becomes especially critical when you have a narrow or fixed window of opportunity in which to operate (i.e. you’re running a promotion that lasts just a few days, you just introduced a new product, it’s a holiday sale, or a store just opened).
- SCALE. The power of the cloud enables retailers to quickly crunch high volumes of data across dozens of sources. When more questions can be asked of the data and more people are empowered to discover the answers for themselves, the quality and speed of insights grows exponentially. Most marketing and merchandising platforms present data in aggregate and don’t allow users to dig into data at a granular level. But the ability to slice cohorts down to SKU level for instance often yields the most surprising and impactful insights.
- EXPLORATION. Every cohort analysis should start with an educated hypothesis, but identifying your highest value cohorts requires trial and error. Rapid iteration and data exploration is necessary to create, discover, and refine cohorts based on an unlimited combination of data points. This requires choosing a solution with a user interface that is easy to learn and use, regardless of technical expertise.
Once you have identified your best and worst-performing cohorts based on these metrics, you can begin further analyzing and grouping them by the profile data points you collected in Step 2. Do customers within these groups purchase the same or similar products? Do they come from the same lead sources? Do they share particular demographics such as age or education level?
It’s important to look at both good and poor-performing segments to reconcile any similarities between the two. For example, customers with the lowest acquisition cost may come from Twitter. However, customers with the lowest lifetime value may also come from Twitter. Therefore, it doesn’t make sense to focus all of your ad dollars on Twitter.
6. Optimize your marketing and merchandising efforts
Armed with a detailed, accurate, and up-to-date picture of your most valuable customers all the way through their journeys, you can begin targeting prospects with similar profiles using highly relevant messages. You can see where opportunities lie to drive upsells, cross-sells, and additional purchases through more personalized product recommendations. What’s more, you can identify the types of products these high-value customers purchase to optimize your inventory strategy, product mix, and placements.
Sample scenario: Your team decides to allocate more marketing budget toward digital advertising and begin promoting product category pages like “Fishing Equipment” and “Automotive Tools.” But after a few months, you notice customer retention rates are dropping.
After segmenting your customers by conversion path, you see that retention rates are higher for shoppers that come in through the individual product pages vs. those that come in through the product category pages. Drilling down even further, you discover that shoppers who enter through the individual product pages and then watch a video about the product convert 20% faster.
As a result, your team reallocates more of your budget toward steering people to individual products instead of general product category pages. Additionally, you create product demo videos and crowdsource user-submitted product videos that autoplay at the top of each product page to increase conversions.
Target segment analysis success story
Lovepop is an innovative company that designs amazing pop-up cards, flower arrangements, and other gifts that are perfect for celebrating holidays, milestones, and everyday moments. It’s critical for Lovepop to optimize their inventory to ensure the best products are shown to site visitors.
That’s why they turned to Sigma to help them get the most value out of their data and optimize merchandising and inventory management. “We use Sigma to quickly surface information about our inventory position, which enables our sales and planning teams to make the best decisions possible,” says Tophi Rose, Director, Analytics & Data at Lovepop.
Sigma is helping the Lovepop team analyze product affinity — figuring out which products, and which aspects of those products, are increasing conversions. “At the end of each season, we use Sigma to create a detailed report to assess which product types and characteristics were successful,” explains Rose.
We use Sigma to quickly surface information about our inventory position, which enables our sales and planning teams to make the best decisions possible.
Tophi Rose Director, Analytics & Data at Lovepop
“For example, we have had a lot of success with our flower bouquets. By understanding how well this product category is doing, we can justify investing more resources into developing additional products within that line.” Sigma also helps the team at Lovepop optimize which products are recommended to visitors on their digital storefront, leading to increased sales and higher customer satisfaction.
The Future of Retail: Clearer Targets, Personalized Recommendations, Greater Profits
Target segment analysis is a proven play every strategic marketer and merchandiser should have in their quiver to effectively narrow their aim, reach the most profitable audiences, and drive the best product strategies for their company.
The ability to understand and track market changes and adapt in near real time is sure to put your retail business leaps and bounds ahead of the competition!
Let’s Sigma together! Schedule a demo today.