What Roles Will Exist In Data Teams In 2030?
Table of Contents
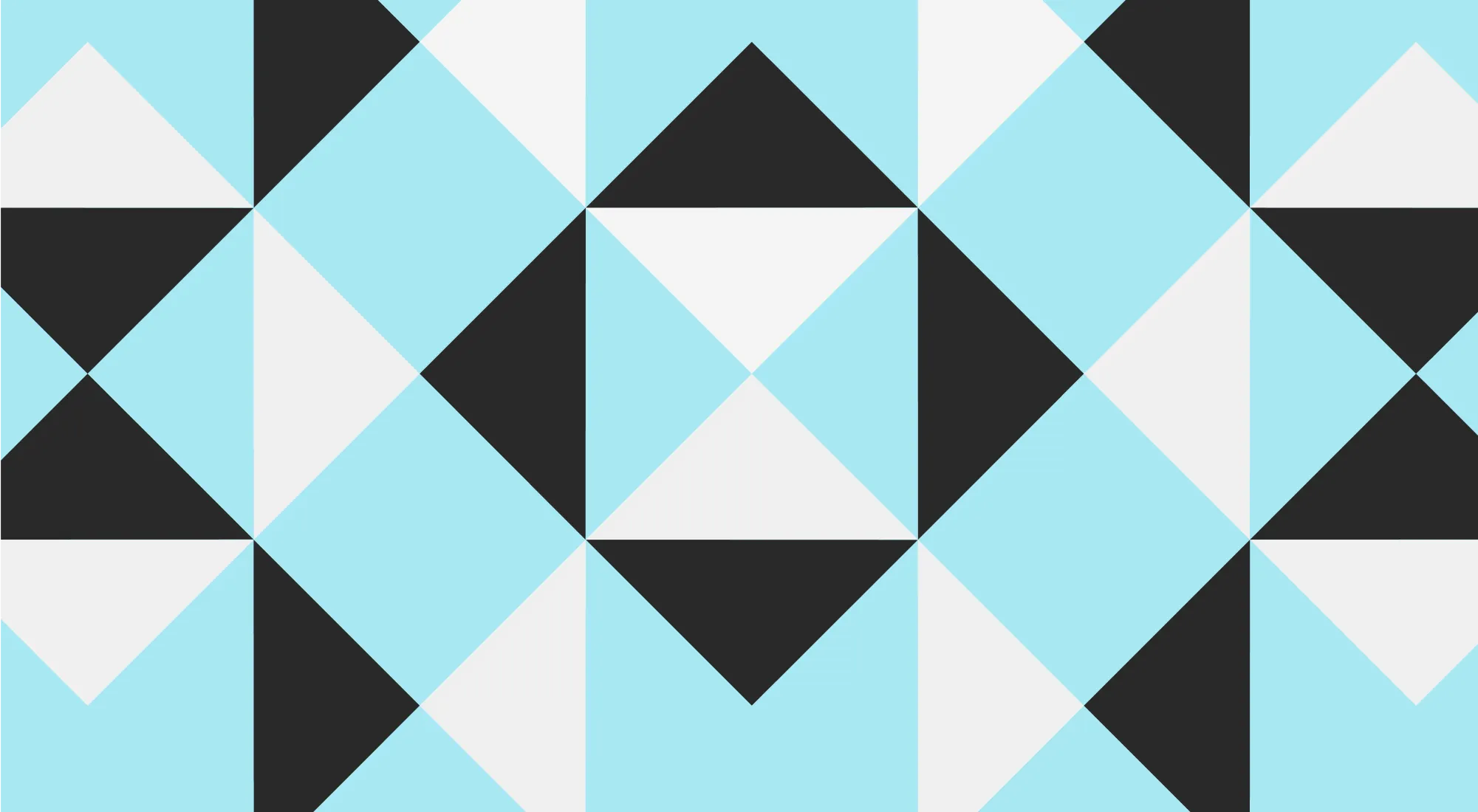
Organizations have never had more information at their fingertips, yet they have more questions about how to utilize it. The volume of data from apps, sensors, transactions, and interactions continues to climb, but the structure of many data teams hasn’t kept pace. Most companies still organize around traditional roles where engineers build pipelines, analysts pull reports, and business leaders try to make sense of what arrives in their inbox.
The next few years will bring a different kind of demand. As automation takes over more repetitive work and business expectations rise, organizations will need more than technical specialists moving data from one place to another. They’ll be looking for teams that think like product managers, work across disciplines, and help turn raw information into insights the business can act on with confidence.
Preparing for this shift means more than adding headcount or adopting the latest tools. It calls for rethinking what a high-impact data team should look like and building it with intention. For leaders shaping their organization's approach to data, the road to 2030 starts now.
How the data team has evolved (and why it matters)
Not long ago, data lived behind carefully guarded walls. Specialized teams controlled every query, every dashboard, and every request for information. Analysts relied on engineers to unlock datasets, while business users relied on analysts to interpret the results. It was a system designed for control and caution: a structure that prioritized accuracy over speed, and predictability over flexibility.
As companies relied more heavily on data for their daily decisions, cracks in the old structure became increasingly difficult to ignore. Layers of handoffs separated questions from answers, slowing down the insights leaders needed to move quickly. Analysts often found themselves stuck in queues, waiting for engineering support to access or transform even basic datasets. Business users also grew frustrated, as opportunities to act on the information passed by. To break through the bottlenecks, organizations began embedding analysts directly inside business units, giving teams more autonomy to explore, build, and act on data without waiting for approvals.
This shift reshaped what it meant to work with data. Running reports was no longer enough. Collaboration moved to the center of the work, along with the need for faster insights and a tighter connection between business goals and data strategy. The old centralized model didn’t disappear overnight, but it stopped being the default. For leaders building teams for 2030, this change presents an opportunity to reassess what skills and roles are truly necessary. Here’s some more ways technology is shaping the data career landscape.
What new skill sets are shaping the modern data professional
Hiring for technical skills alone no longer covers what organizations need. Future-ready data teams require people who can connect insights to decisions and understand how information moves through the business, not just how to process it. By 2030, the most successful data professionals will do more than pull reports or optimize queries. They’ll help shape how information is gathered, interpreted, and turned into outcomes that move organizations forward.
One of the most important shifts is the rise of data storytelling. The ability to frame insights in a way that leaders and frontline teams can utilize has become a defining skill. Storytelling with data isn’t about making slides prettier; it’s about giving context, nuance, and meaning to the numbers. User experience thinking is becoming just as important.
Data teams are no longer building dashboards for other analysts; they’re building for marketing leads, finance directors, and operations managers who may not think in SQL or schemas. Designing clean, intuitive tools that make information accessible without extra training is quickly becoming a baseline expectation.
Governance fluency has also taken center stage. As data privacy regulations tighten and public scrutiny intensifies, professionals who understand how to build pipelines while also protecting, labeling, and documenting data responsibly are becoming increasingly essential across various industries.
Beyond governance, platform and integration awareness are rising in value. Understanding how modern data platforms interconnect and how to integrate different systems across departments is now crucial for creating environments that scale without constant friction.
Soft skills
The soft skills side is evolving as well. Strategic problem-solving is becoming a bigger differentiator for analysts and engineers who can tie their work directly to business outcomes. Cross-functional communication: the ability to navigate between technical teams and business leadership without losing either group along the way is mandatory for teams aiming to move quickly and cohesively.
Technical depth still matters. However, the teams that will thrive over the next decade will be those that balance engineering precision with business strategy, human-centered design, and an instinct for asking the right questions, not just answering them.
How will automation and AI affect the human side of data work
Automation is already changing what data teams spend their time on. Many of the most repetitive tasks, such as moving data between systems, formatting datasets, and generating basic reports, are shifting toward automated workflows. Instead of replacing people, these tools are opening up more space for teams to focus on the work that requires human judgment: asking the right questions, connecting insights across departments, and communicating findings in ways that move the business forward.
As AI models become part of standard analytics workflows, new responsibilities are emerging too. Teams are being asked to guide AI use thoughtfully by reviewing model outputs, questioning biases, and shaping how machine learning fits into broader business strategies. It’s a shift that rewards curiosity, cross-functional thinking, and ethical decision-making just as much as technical ability.
Tools like Ask Sigma are helping make these transitions smoother by weaving AI directly into everyday analysis. Instead of forcing teams to learn entirely new systems, Ask Sigma lets users explore their data using natural language, all within the familiar workbook environment. Combined with Sigma's broader AI Toolkit, teams can automate common tasks, surface deeper insights, and stay firmly in control of the decisions they drive.
The future of data work won't belong to those who automate the most. It will belong to teams that know when to automate, when to challenge, and when to dig deeper.
The rise of analytics product managers
As data shifted closer to the business, a new type of role quietly started gaining ground: the analytics product manager. Instead of building dashboards on request, these professionals think more like product owners. They manage the entire lifecycle of a data tool or platform, shaping what gets prioritized, ensuring that solutions meet real user needs, and monitoring long-term adoption.
This mindset is a major departure from traditional project management. Instead of focusing solely on deadlines or deliverables, analytics product managers stay tuned into how their "products" actually perform over time. They track user satisfaction, drive iterative improvements, and bridge the gap between technical teams and business objectives.
Launch dates matter, but they aren’t the only measure of success. What matters more is whether the tools built genuinely help people make smarter, faster decisions. The role demands a blend of technical fluency, business strategy, and a deep understanding of how real users interact with data.
Organizations that invest in this role are starting to see better returns on their analytics platforms, with fewer abandoned dashboards and more business-aligned outcomes. Salaries reflect the growing demand, with U.S. analytics product managers earning an average base salary of approximately $131,127.
Data stewards and compliance officers
As more teams rely on data to drive decisions, the cost of getting it wrong has climbed. Data stewards and compliance officers are becoming increasingly central figures in data organizations, as they play a crucial role in meeting regulatory requirements and protecting the integrity of the work. They oversee how information is collected, labeled, stored, and shared, ensuring that privacy rules are followed and that users across the business can trust what they see.
Companies of all sizes are under pressure to treat data responsibly, whether it’s customer behavior, employee records, or internal analytics. Strong stewardship protects more than compliance checklists. It strengthens the credibility of every dashboard, report, and model built on top of that data.
Salaries are starting to reflect the increasing importance of these roles. Data Stewards in the U.S. typically earn between $54,000 and $121,000, with an average salary of around $78,870. Compliance Officers who specialize in data privacy see similar compensation, with salaries ranging from $51,000 to $130,000 and an average of $80,088.
Consider hybrid data and business roles (that blend business strategy and engineering)
As data becomes an integral part of every business decision, the clear divisions between technical and strategic work are starting to blur. New hybrid roles have emerged to bridge the gap, helping organizations turn insights into action more quickly and with fewer misunderstandings along the way.
Analytics engineers, for example, build and maintain the data models that analysts and business users rely on, ensuring that the numbers displayed in dashboards accurately reflect reality. Their compensation reflects their growing importance, with analytics engineers in the U.S. typically earning between $113,625 and $141,152.
Beyond engineering, roles such as data translators and operations-minded analysts are gaining traction within organizations. While they may not always have formal titles, these professionals focus on making technical insights understandable, actionable, and closely tied to business goals. Their value is evident in project outcomes and the smooth communication and execution of teams against shared objectives.
These hybrid functions are reshaping what it means to "work in data," blending engineering precision with strategic thinking and redefining the skills companies need to prioritize when building future-ready teams.
Additional roles to consider for future teams
Beyond the emerging roles within teams, leadership positions related to data are also evolving. Chief Data Officers (CDOs), once focused mainly on compliance and governance, are increasingly expected to drive business value through innovation and strategic partnerships. They're becoming architects of growth initiatives that rely on deeper, faster, and smarter insights. Salaries reflect the expanding expectations, with CDOs in the U.S. earning between $230,000 and $430,000.
Meanwhile, as AI continues to mature, new specialties are emerging. AI Product Managers are emerging to oversee machine learning initiatives from a business outcome perspective, ensuring that models serve strategic goals instead of becoming isolated technical experiments. Prompt Engineers, a relatively new title, are helping teams design, refine, and maintain the inputs that drive large language model performance. These roles aren’t replacing traditional data work. They’re layering new capabilities into teams that still rely heavily on human judgment and collaboration.
For data leaders considering the future, these shifts signal a broader trend: building a team is about shaping a workforce that can think strategically, adopt new tools thoughtfully, and connect insights back to business outcomes.
Preparing your organization for the data team of 2030
Building the data team of 2030 isn’t about predicting every new technology or chasing every trend. It’s about shaping a workforce that thinks flexibly, adapts strategically, and stays focused on the business outcomes that matter most.
That means looking beyond technical resumes and hiring for curiosity, cross-functional fluency, and the ability to connect insights to real-world action. It means creating environments where data stewards, product managers, hybrid analysts, and AI specialists can work side by side without getting lost in translation, and recognizing that leadership is about setting the tone for how data is treated, challenged, and championed across the organization.
Teams that invest in these shifts now will be better prepared for whatever comes next. And if you’re thinking about what your next step might be, know that companies like Sigma are already building the future of analytics. Explore careers at Sigma and see how you can be part of shaping what’s ahead.