From Data to Decisions: The Power of Leading a Data-Driven Organization
Table of Contents
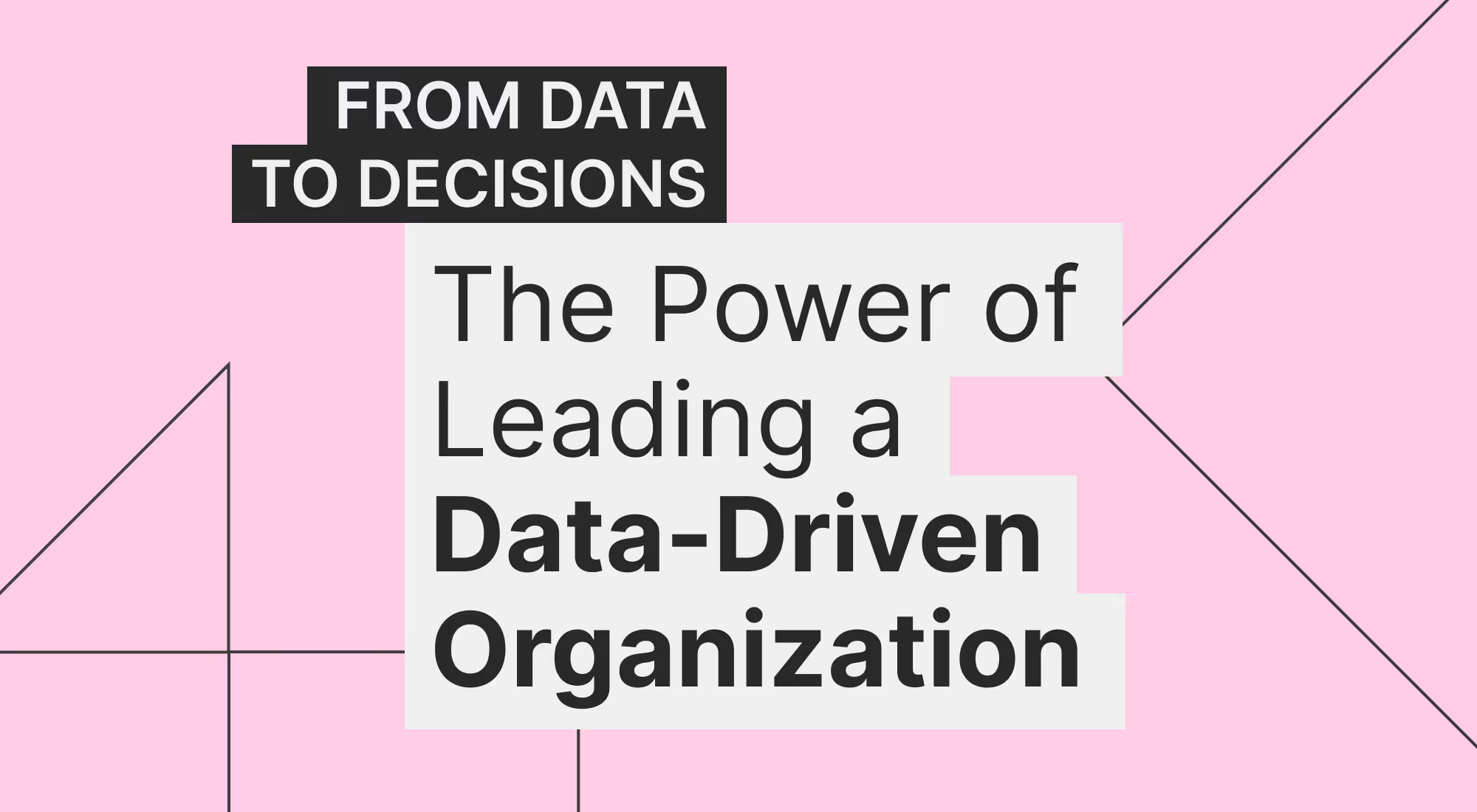
In today's fast-paced world, being data-driven isn't just a trend—it's essential. Companies are using data to make smarter decisions, streamline operations, and innovate faster. Sigma's advanced analytics and business intelligence put us at the forefront of this transformation.
Understanding a data-driven organization
A true data-driven organization isn’t just about having big data; it’s about making data a core part of every decision. With Sigma, you don’t just access data—you embed it into every aspect of your business. Our platform integrates seamlessly across all your systems, providing a unified view that powers strategic decisions. Real-time data and actionable insights from Sigma set your business apart, driving success like never before.
Key attributes of data-driven organizations
Data-driven organizations stand out with three key traits: Robust data infrastructure, a dedication to data literacy, and a culture that champions data-driven decisions. Sigma fuels these strengths with:
- Comprehensive data integration: Connect disparate data sources into a cohesive, actionable dataset.
- Advanced analytics: Use Sigma’s powerful analytical tools to uncover deeper insights.
- Real-time decision making: Access and analyze live data to stay ahead of the market curve.
The journey to data maturity with Sigma
Starting your data-driven journey? Let Sigma guide you through every stage of maturity:
Initial data collection
Kick things off with Sigma by integrating and consolidating data from all your sources. At this crucial first step, we make sure your information is not just accurate but easily accessible. Sigma effortlessly imports data from CRM, ERP, and other operational tools, creating a central hub that eliminates inconsistencies. This solid foundation is key to building reliable analytics that power every phase of your data evolution.
Departmental analytics
As Sigma spreads across departments, it dismantles traditional data silos. Marketing, sales, and operations teams can customize Sigma’s features to meet their specific needs, fostering collaboration and boosting departmental analysis. This integration aligns perfectly with the overall business strategy, making your organization more agile and responsive to market shifts.
Enterprise-wide analytics
At this level, Sigma becomes your organization’s central analytics hub. It supports complex data analysis and predictive modeling, giving you a comprehensive view of your company’s data to drive strategic decisions. With Sigma’s advanced analytics, including AI and machine learning, you can anticipate trends and automate insights, streamlining operations and sharpening your competitive edge.
Data democratization
Sigma’s user-friendly interface makes data accessible to everyone, regardless of technical expertise. This empowers all employees to engage with data and contribute to insight-driven discussions. By broadening data access, Sigma speeds up decision-making and boosts individual accountability, creating an inclusive environment where data-driven insights are integral to everyday business operations.
Data culture
Sigma fosters a strong data culture where every decision is anchored in robust analysis. This cultural shift represents a universal commitment to leveraging data as a key asset for business success. Sigma enhances data visibility and communication across the organization, embedding data into strategic planning, operational adjustments, and continuous improvement efforts, ultimately elevating both efficiency and strategic agility.
How to transform your organization into a data-driven powerhouse
Transforming into a data-driven powerhouse isn’t just a strategic move—it’s a full-scale revolution. Here’s how to navigate this crucial shift:
Set clear data goals
Define what data-driven success looks like for your organization. Pinpoint goals like enhancing data accuracy, accelerating analysis, or boosting customer insights. These objectives will guide your data initiatives and help you measure progress.
Invest in the right tools
Equip your team with cutting-edge analytics tools. Sigma’s platform delivers powerful analytics that are accessible to everyone, whether they’re tech-savvy or not. The right tools empower your team to unlock valuable insights from complex data.
Cultivate data literacy
A thriving data culture demands robust data literacy across all levels. Invest in training that demystifies data concepts and analytics processes for your employees. Customize this education to fit various roles, ensuring everyone can contribute meaningfully to data-driven discussions.
Promote data governance
Implement solid data governance policies to ensure quality and security. Effective governance maintains data accuracy, consistency, and access control. This foundation is vital for building trust in your data and the insights you derive from it.
Foster a culture of experimentation
Encourage experimentation and innovation with data. Allow your teams to explore new ideas without fear of making mistakes. Experimentation can lead to discoveries that propel your business forward and instill a sense of ownership and engagement among team members.
Use data to drive decisions
Make data and analytics central to your strategic decisions. Don’t just collect data—actively use it to guide your choices. Over time, this practice will become second nature, reinforcing the power of a data-driven approach.
By embracing these strategies, your organization can evolve into a data-driven leader, leveraging insights to drive efficiency, innovation, and competitive edge.
Unlocking the advantages: Why becoming a data-driven business is essential
Embracing a data-driven approach is a game changer for your business. Here’s how going data-driven with Sigma can transform your organization:
Enhanced decision-making
Forget intuition—make decisions backed by data. With Sigma, you’ll minimize risks, boost strategic planning, and respond to market shifts with confidence.
Improved operational efficiency
Spot inefficiencies and refine your processes. Optimize supply chains, cut downtime with predictive maintenance, and automate routine tasks. Data insights lead to cost savings and operational excellence.
Increased customer satisfaction
Dive deep into customer needs with data analysis. Deliver personalized service and marketing, and boost loyalty. Tailor your offerings to what your audience truly wants.
Innovative products and services
Fuel innovation with a data-driven mindset. Spot new opportunities and trends before the competition. Create products and services that meet evolving demands and position your company as an industry leader.
Scalability and growth
Leverage data-driven insights to support scalability. Understand what works and what doesn’t, allowing you to allocate resources effectively, scale operations efficiently, and grow sustainably without the trial-and-error approach that often hampers expansion.
Risk management
Improve risk management with advanced data analytics. Predict potential issues and proactively address them, from financial risks to operational disruptions. Maintain stability and ensure business continuity by being preemptive rather than reactive.
Empowered employees
Empower employees at all levels with access to data. Enable quick, informed decisions, leading to higher job satisfaction, increased productivity, and a more dynamic workplace culture that encourages data-driven initiatives.
The shift to a data-driven business with Sigma might take effort, but the impact is undeniable. Transform your operations and set the stage for long-term success.
Implementing Sigma: First steps
Embarking on your data-driven journey with Sigma means strategic planning and engagement from all levels of your organization. Begin with a pilot project to show Sigma's immediate value, then gradually expand its use as familiarity and confidence grow.
Why choose Sigma?
Choosing Sigma for your data analytics needs transforms how your organization handles data, offering numerous advantages:
User-friendly interface
Sigma’s intuitive interface is designed for everyone, regardless of their technical expertise. This ease of use fosters a more inclusive data culture, making it simple for everyone in your organization to engage with and benefit from data.
Scalable infrastructure
As your organization evolves and your data needs grow, Sigma scales effortlessly with you. Our infrastructure handles larger datasets and more complex queries without missing a beat, so your analytics capabilities keep pace with your ambitions.
Security and governance
Sigma emphasizes security and governance, protecting your data against unauthorized access and breaches. With comprehensive compliance tools and protocols, Sigma helps you meet industry regulations effortlessly, maintaining your data's integrity and confidentiality.
By implementing Sigma with a strategic approach and understanding its distinct advantages, your organization can leverage powerful analytics to drive decision-making, innovation, and growth.
The data-driven edge
In today’s landscape, being data-driven isn’t optional—it’s essential. Sigma equips you with the tools to transform your organization into a beacon of data-driven excellence. Embrace Sigma and lead with data as your strategic advantage.